How to simulate an entire operation before productionCreating a simulation, or “digital twin,” of a plant or piece of equipment can help operators prepare for any eventuality
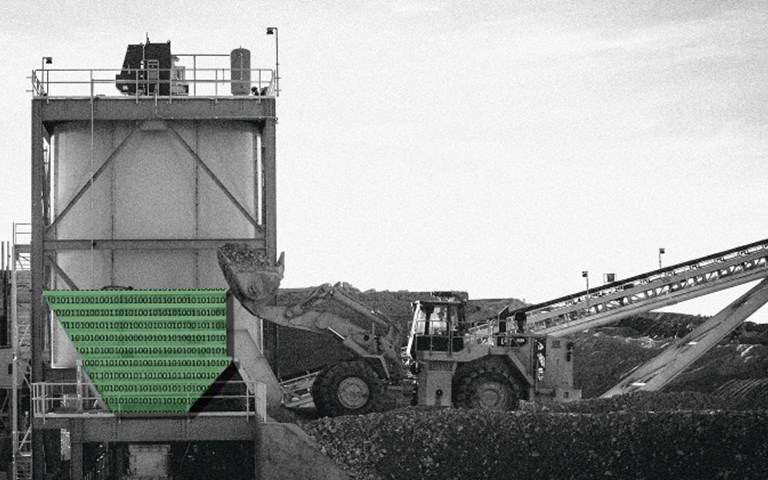
In November 2010, the first documented use of the phrase “digital twin” appeared in a technology roadmap by NASA. The agency envisioned simulations so true to actual vehicles or systems that a mission could be flown virtually in its entirety before it launched. Digital twins would be based on physical models, sensor updates, fleet history and other data sources. They would help test different parameters and flight plans, provide continuous health predictions based on real-time operating data and guide troubleshooting.
Since then, the term has found its way into manufacturing and, eventually, into mining. Sohail Nazari, business development manager at Andritz Automation, explained that there is no official definition of digital twins for process industries. “However, there are three important aspects that are common throughout various descriptions,” he said. “When simulation is a core functionality of the process, along the entire life cycle of the plant, with direct linkage to operation, that process has a digital twin.”
Some of the data that might be used to model a process plant during the design phase, for example, include the pump specs, plant elevation, the length of the pipes in the plant, and mineral characteristics sourced from a licensed database. How these variables will affect operations is predicted by models that rely on first principles of physics and chemistry. Project consultant William Thomas, who has used the technology in project design for Hudbay Minerals and other clients, said an iterative process adds more detail from the initial project assessment through the detailed design. Once the plant is running, operating data is fed back into the model. Some mines that have been operating for long enough to have years of historical data – ranging from weather conditions to drill and blast settings – can use that to build a simulation using machine learning.
According to Anderson, the digital twin can give more immediate feedback on whether any given setting change is going to help or hurt. Original photo courtesy of OceanaGold
Digital twins at the Haile gold mine
Andritz has offered digital twin technology to the mineral processing industry for offline applications for several years, however the company’s first customer for its real-time online digital twin offering was OceanaGold’s Haile gold mine in South Carolina, which started up in 2017. “We have the same mindset that we’re both looking to try newer things,” said Caelen Anderson, metallurgical superintendent at Haile. “We’re trying to take our plant and fully automate it, effectively. From crushing all the way to the tail end of the plant.”
Part of that vision is the digital twin, which Haile is just beginning to integrate into operations. “We have a digital twin on our cyclone feed line that they’ve been tracking for about a year,” said Anderson, “and that was more of a proof of concept to really show it’s reliable and we can use it.” In August, Andritz and Haile began tuning the model using operating data. They recently added digital twins for the cyclone underflow and overflow as well.
According to Anderson, the digital twin can give more immediate feedback on whether any given setting change is going to help or hurt. “Right now I’m relying on guys to go out there manually and sample every hour,” he said. “But when you’re operating the control room and you’re making changes to feed densities and pressures, you’re not seeing the effects immediately when you’ve only got an operator doing it once an hour.”
Related: OceanaGold writes the latest chapter in the long history of South Carolina’s Haile mine
It also helps indicate pump performance by alerting operators when actual data differ too widely from model predictions. “If your pump is running at a certain speed and a certain power, and you know the slurry density, you should be putting out X amount of volume from the pump,” explained Anderson. “If you see that performance start to drop off, it’s a great indicator that something might be wrong with your pump. This can save a lot of time and money by keeping minor failures from turning into major ones.”
Anderson said he thinks the benefits substantially outweigh the costs. “If my cyclones start to have issues, and I’m alerted by this soft instrument, that’s going to save me from some pretty significant plant downtime, reduced tonnage or opportunity lost, just by getting a guy up there immediately,” he said. “It can pay for itself very quickly.”
Andritz and Haile are also working on monitoring the thickener underflow. “If you lose that instrument, you are kind of vulnerable to upset,” said Anderson. The digital twin helps to check the density meter’s accuracy, takes its place in automated operations when it fails, monitors the pump’s impeller condition, and detects sanding in the pipe (whereupon an alarm goes off and the system temporarily increases the pump speed to wash out the sanded slurry).
A growing field, and a caveat
Penny Stewart, managing director of Petra Data Science, divides her company’s simulation solutions into four categories: drill and blast, geometallurgical prediction, process control and reliability engineering. In one scenario, historical drill and blast data can be used to simulate how changing the burden and spacing of blast holes will affect SAG mill throughput. “By bringing the holes closer together, then they’re getting a small uplift [in throughput],” Stewart said, demonstrating a digital twin created for PanAust’s Ban Houayxai mine in Laos. “I think it’s two per cent; on a mine site where your SAG mill is the bottleneck, it directly translates into two per cent more cash out of that operation.”
Over the past few years, Petra has developed machine learning models for simulating how geology affects a processing plant. The digital twin integrates 3D geological data with time series processing data. Each new accumulation of data is fed back into the model, building its depth and breadth over time.
Stewart said she thinks this is a substantial improvement over pilot-testing rock samples. “The sample size is tiny with respect to what you’re going to put through that plant over the life of the mine,” she said. “This is where companies [run into problems] because they think they’ve done a decent job of predicting with these samples, but then they go through areas that they haven’t sampled or that aren’t captured well in the empirical model. They get results that are, let’s just say, undesirable outcomes.”
At GE Transportation, the Digital Mine team is using digital twins for asset and operation performance management, drill guidance, and collision awareness. Kevin Shikoluk, global strategic marketing leader for Digital Mine, explained the difference between predictive maintenance and digital twins: “Asset performance management could predict that you have an issue with a pump such as corrosive slurry in the pump, or you may have a valve that’s going to break down,” he said. “The digital twin will show you what happens if you don’t self-correct, or if you don’t fix the issue in the pump. The digital twin allows you to play with level settings in real time before you execute in the plant.”
Shikoluk said that about a third of GE Transportation’s Digital Mine customers are using digital twin concepts and strategies. “They could be trying to digitalize an asset, or they could be digitalizing their entire plant’s operation,” he said. The first step is trying to decide where to collect data, how often, and what data should be collected. Then GE and the customer need to agree which parameters to analyze and how to define acceptable ranges.
In April 2017, GE and Perth, Australia-based miner South32 announced a three-year deal, nine months after South32 paid a visit to GE’s MINExpo booth. “With South32, we were successful because we were able to would isolate specific assets that we would want to demonstrate success on, and then once we were able to demonstrate success on a small subset of assets, we would scale to the plant and operations level,” said Shikoluk.
For an industry with a conservative reputation, the rate of adoption that GE has seen is impressive. However, in Thomas’s experience as a consultant, there is substantial resistance to trusting a digital twin to design new facilities – not just because of conservatism, but also because it could reduce work for EPCMs. “The problem is, the whole industry makes money by man-hours,” he said. “After I gave this PowerPoint presentation asking [an EPCM employer] for more computers to do this stuff on, one of the VPs at the end said, ‘So what you’re telling me, Mr. Thomas, is if you use this technology, we can design a plant in half the time for half the money.’ I said, ‘Yup.’ You know what he said? ‘You’re out of your effing mind. I make money by charging hours.’”
"Once we build a fully dynamic digital replica of the plant, we can use it as an operator training simulator, the same way a flight simulator works." -- S. Nazari. Original photo courtesy of OceanaGold
Accuracy and uptake
Digital twins need to be accurate and reliable if they are to be trusted. Anderson said his initial reaction to the idea was skeptical. “But we’ve had the virtual instrument in our cyclone feed running for well over a year, and it’s close!” said Anderson. “Like, really close. Just yesterday, when I had one of the guys from Andritz here, we were actually running our entire circuit based off of a virtual instrument.”
The effect of tuning to operation data is obvious. In one cyclone feed density data sample shared by Nazari, adding ten days of operating data to the model brought its difference from physical instrument measurements to one per cent RMSD from two per cent. (RMSD is root-mean-square deviation, found by squaring the difference between each set of data points, averaging them, and taking the square root of the average.) “Bottom line, the most important thing is the fidelity of the models,” said Nazari. “Fidelity means how accurate the digital twin represents the reality. At Andritz, we ensure fidelity by combining first principle models with whatever data is available, including chemical, mechanical, metallurgical, and control.”
At the end of August, Anderson’s operators had not yet seen the digital twin in action and still needed to buy in. “In my job, that’s probably one of the biggest challenges I have,” he said. “They’ve been running the plant manually for their entire lives, and I come in and say, ‘Hey, you know this thing that doesn’t exist? Well, we’re going to run the plant based off that now.’” But Anderson thinks his operators will likely be won over.
From static to real-time simulation
Although historical data from an operation are useful, they are not strictly necessary for building a digital twin. Andritz’s IDEAS simulator was first used to optimize feasibility studies on new projects.
“A lot of times, the plant is not even built yet, so there’s no operational data available right now,” said Nazari. “As we go along, we incorporate the information that is available. For example, we start with the models for the SAG mill based on manufacturing information, and we add information as the breakage functions are available from the lab samples.” Andritz’s digital twin technology has many applications, from automated circuit design at the pre-feasibility stage to detailed piping and instrumentation diagram validation to decrease cascading cost in detailed engineering. “Once we build a fully dynamic digital replica of the plant, we can use it as an operator training simulator, the same way a flight simulator works. Once the plant is operational and running, we connect these high fidelity models to real-time operation.”
Thomas takes issue with EPCMs and equipment manufacturers over-designing plant capacity to be on the safe side. He argued that precision in modelling can cut down on capex and related opex, and sometimes save a potentially economic project from rejection. “The bottom line is that most facilities are at least 125 per cent more expensive than necessary to meet the initial business case,” said Thomas. “This needs to be addressed.”
Better computer power and communications technologies have allowed simulations to incorporate real-time operating data, expanding the ways they can be used. If the simulation is accurate enough, it can fill in missing data when a sensor malfunctions, and highlight problems if there is a discrepancy between actual and virtual measurements. In real-time installations, multiple simulations are running a number of different what-if scenarios parallel to operation.
Related: Transitioning from information overload to efficient operation with short interval control
There are still quite a few hours spent integrating this new technology. Right now it takes about six to eight weeks to integrate a new mine’s data into the digital twin for mine value chain optimization that Petra markets as MAXTA, for example. Stewart said she expects that integration period to become shorter as partnerships and collaborations with other service and software providers further standardize the data required for the model. “What was an eight-week requirement might drop down to a few weeks.”
The company is working with Maptek to integrate with its 3D mine planning software. “There’re lots of other companies that do this same thing and we’re just as open to talk to any of them,” said Stewart. “But this company actually approached us and said, ‘Would you like to collaborate to do this?’ And I said, ‘Yeah, that’s a great idea.’ Because what that means is a mine planning engineer can evaluate the mine schedule in the MAXTA digital twin and see what effect it has on that processing plant.”
Work in progress
Some measurements and processes are easier to simulate than others. For Nazari, the “holy grail” of comminution is the virtual measurement of the mill charge. It would help to further optimize SAG mill performance, but the physical sensors in question are not available.
At GE, said Shikoluk, digital twins are strong at the asset level, but creating a digital twin of an entire plant’s process, such as smelting or refining, is a “roadmap” item. “We have the ability to create digital twins of components of mining sites,” he said. “We don’t have the ability, nor does the competition, to create a digital twin of an entire process or operation.”
There are also many questions that could be fruitfully addressed by future research. For example, when operations move to a new area of the mine, will a model built on historical data continue to be useful? In collaboration with industry partners, Petra is currently applying for grant funding from the Minerals Research Institute of Western Australia to study just that. She hypothesizes that the models will still be applicable because they are based on intrinsic properties of the rock – geochemistry, percentage of different elements, what minerals are present. That is in contrast to more traditional subdivisions into Rock Type A, Rock Type B, and so on.
Nazari remarked that historical operating data are better at describing normal conditions than abnormal conditions, anyway. “You need those models to be able to react when your densometer or your pump fails,” he said, “but those do not happen too much, so you don’t have that much data for that. If we build [models based on first principles], those models are valid not only for normal conditions, but for abnormal conditions.”
Manufacturing as a model?
The European Union is working with Siemens on a research project to develop digital twins in manufacturing. However, Stewart said she believes this project will be of less value for mining than NASA’s research because it excludes spatial grid-based data simulation. “A lot of the variability in how a processing plant performs is related to the spatial variability in the rock,” she said. “Whereas in manufacturing, you don’t have that intrinsic variability because you’re dealing with known quantities [that are] very well understood from a physics-based point of view.”
Stewart said that linking spatial mineralization to time series process data is complicated, because the ore needs to be tracked on its journey to and through the processing plant – something she says her company’s software is able to do.
Asked about the effects of ore variability, Nazari answered that it depends on the application. “The ore body doesn’t have any influence on a densometer, for example, because we use a dynamic model of the density based on the pump’s power consumption, pressure, and things like that,” he said. But it does influence the virtual instrument for finding p80 and the SAG mill charge.
“We try to make it independent from the variables that are out of our control,” said Nazari. “If independence is hard to achieve, we require additional information.”
In any case, the existing uses have proven themselves – in some quarters, anyway. “It’s really amazing technology,” said Anderson. “I was probably one of the more skeptical people about it. But now that I’ve seen it, and I’ve seen what you can do with modelling around the plant, I’ve been pretty impressed.”
More Technology
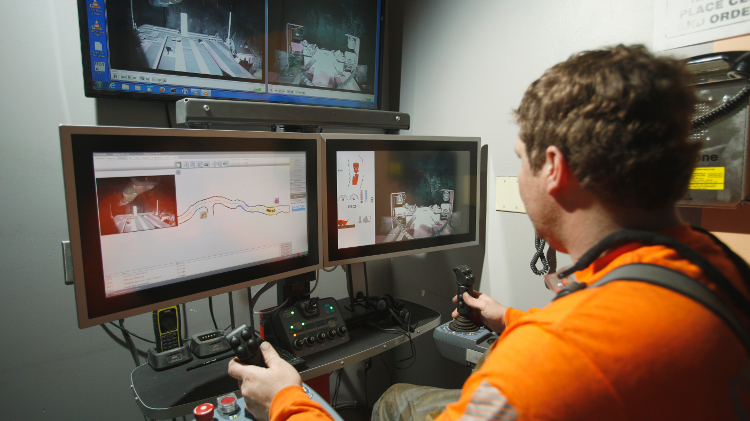
The automation revolution
There is no doubt the future of mining is automation. But what does that look like, and how will we get there?
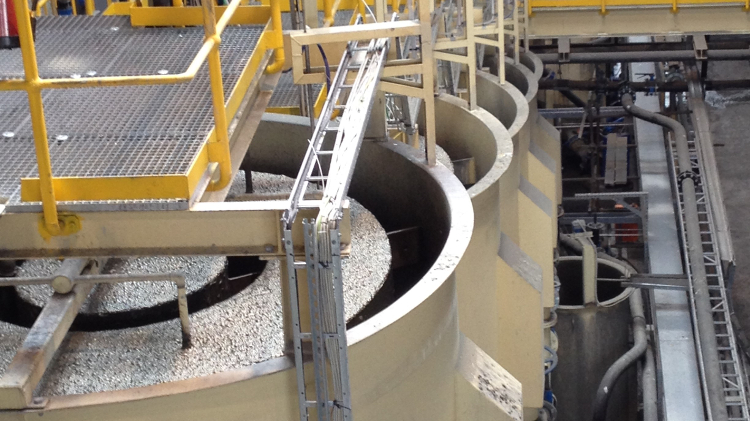
The future of flotation
Buoyed by pressure to cut costs and improve recovery, new flotation technologies are on the rise