Teck Resources has added machine learning to its mobile equipment monitoring to help better identify patterns that may predict failure. Courtesy of Teck Resources
Teck Resources recently launched a machine learning initiative to improve its preventive and condition-based mobile equipment maintenance program. Goldcorp is currently speaking with potential partners and planning to do the same in the very near future. There is a lot of buzz in the mining industry these days about the potential for artificial intelligence applications for maintenance of mobile equipment.
“Two years ago, not many people were asking about machine learning and predictive analytics,” said Colin Donnelly, vice-president of product management at Dingo Mining, a company that provides condition management and predictive maintenance of equipment for mining companies across the globe. “Now it’s the first thing they ask us.”
Miners are hoping new artificial technologies can connect all the onboard and sensor data points on equipment to map out a far more effective approach to condition-based maintenance. It is in its early days still, but the journey to that revolutionary new approach has begun.
Ahead of the curve
Teck Resources has had a mobile equipment monitoring program since 2011 to track the health of its haul trucks at its steelmaking coal operations. “Across our fleets, we collect 1.5 billion data records from [the equipment’s] sensors every 24 hours,” said Chris Stannell, a spokesperson for the company. “The program involves reviewing this data to identify failing components so that maintenance can be triggered earlier than without the program. This reduces costs and increases asset uptime.”
Related:Teck installs first production-scale machine learning system
Now, in partnership with Google Cloud and global IT-consulting company, Pythian, Teck has also deployed machine learning algorithms that scan through millions of data points from its Komatsu 930E haul truck fleet at its Fording River, Greenhills and Elkview steelmaking coal operations in British Columbia’s Elk Valley region.
“The failures that this algorithm identifies are not typically discernible to a human looking at the data,” said Stannell. “To illustrate the point, consider that the system will give various intermittent warnings that come and go as a haul truck is operated. Some of these warnings don’t mean anything on their own; however, the pattern of warnings can indicate if there is a real underlying issue or not. The algorithm makes sense of these patterns and informs us of the risk of failure.”
A complex and moving problem
It is no wonder the industry is hoping software and machine learning algorithms will come to the rescue – or at least play a big part in it. Mobile equipment maintenance is a highly complex and expensive challenge. In a single mine, the costs can easily run in the hundreds of millions of dollars annually, not including the added cost of unscheduled downtime.
The factors that can result in sudden failures are as dynamic as the weather or the ground conditions on a mine site across the seasons. As well, the equipment and many of its components face a ton of potentially damaging pressures and forces every hour of use. As equipment has grown in size, these have multiplied, said Tim Joseph, a mining consultant and associate dean at the University of Alberta’s school of mining and petroleum engineering. Consider the six tires of a haul truck that carry the load of 300-plus tonnes of material in addition to the truck’s weight as it travels down a roadway, bouncing and pitching and rolling. “That movement doubles or triples the impact of the load on the tires,” he said. As a result, component failure fatigue has increased over the last decade and a half.
During the last ten years, thanks to sensor and onboard technologies as well as software tracking systems such as Dingo’s Trakka, the opportunity for condition-based maintenance has opened up.
This approach to maintenance tracks the condition of equipment daily, monitoring temperatures, pressures, fuel emissions and the quality of fluids passing through the system as well as the efficiency of the burn on the fuel. Instead of servicing and repairing equipment based on assumptions and averages that ignore the equipment’s actual condition, mining operations can maintain the equipment’s availability and health based on the actual readings and operating conditions. This opens the door to pre-emptive repairs and greater avoidance of major failures, in some cases.
“Sometimes you can replace a $10 part and save a million dollar failure,” said Jami Dwyer, a consultant and former head of the predictive maintenance project at Barrick’s digital transformation team.
Other times, the savings come from avoiding unnecessary work.
“You don’t have to replace parts automatically after say 8,000 hours,” said Donnelly. ”You can get 20,000 hours from the part if we treat the equipment right and pay attention to the data. And basically make the right decisions.”
To some extent, everyone is doing at least a bit of condition-based monitoring, said Dwyer. But a bit does not get the same results as a comprehensive, condition-based monitoring system, which involves collecting and analyzing a lot of data and records.
Connectivity
One of the most critical tools for an effective condition-based monitoring program, let alone an artificial intelligence one, is network connectivity. Without that, all the valuable data from the sensors and onboard systems cannot be gathered and analyzed for real-time, or at least daily, monitoring.
“For areas where you have intermittent connectivity issues, condition-based maintenance starts with the ability to collect real-time or near real-time data,” said Kirk Petroski, president and CEO of Symboticware, which has developed a telemetry technology that collects data in real-time and can send the data forward when a network connection becomes available, thus eliminating data gaps. He added, “consistent, high frequency real-time data enables condition-based and future machine learning applications.”
There are a number of reasons why Goldcorp is now looking at the possibility of adding machine learning to its mobile equipment maintenance program but, according to Luis Canepari, the company’s vice-president of technology, “ “we now have the backbone and the connectivity in our mines that is allowing us to capture the data from the fleet in real time. We are starting to use telemetry systems in several of our mines that is enabling us to collect sensor data from our mobile fleet, regardless of the manufacturer, which is the foundation for a proactive condition monitoring program.”
Human and machine interface
Dingo does not simply offer tracking and analytical software. It also uses a team of human reliability experts to analyze the data and recommend actions. “The reason we’ve done that is we have had experience providing the software to large mining companies. We saw one flourish and one be in trouble,” said Donnelly. “We found the difference was due to the application of the software. Software doesn’t do anything if you don’t use it correctly to make the right decisions.”
In fact, the most critical tool today for condition-based and predictive maintenance continues to be a human brain, well trained to interpret the sensor data, which contains a far bigger story than just the equipment’s vital signs. Based on onboard sensor data, said Joseph, it is possible to deduct such things as the road conditions and structural fatigue in the equipment and develop strategies to better adapt the operation of even mammoth-sized equipment to avoid failures. “I don’t even have to look at the road. I can get all that from the onboard system,” he said.
 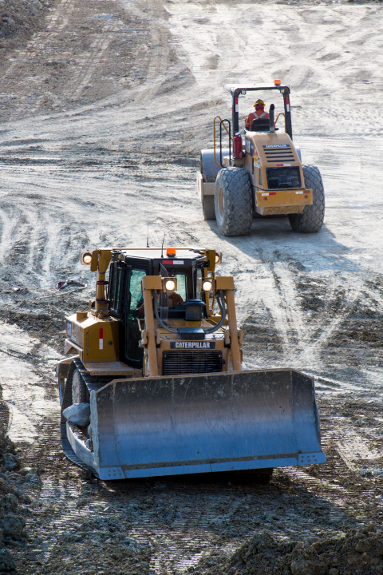 |
As more of a mine's equipment and sensors are connected to a network, the potential to analyze and improve preventive maintenance continues to grow. Courtesy of Goldcorp
|
Effective condition-based management, said Joseph, “is also about examining and tracking the operating conditions,” he said. “The operating circumstances are giving rise to the deficiencies and unknowns when it comes to maintenance. We are looking at not just the machine itself but the operation conditions. The real key is having a very good understanding of interactions between the equipment and the ground.”
Whether it is to develop algorithms or interpret sensor data, Joseph and Dwyer said they believe that taking full advantage of new technologies for condition-based mobile equipment maintenance will require building a better-trained workforce that combines technology and mechanical knowledge.
“We are seeing the adoption of condition-based monitoring technologies at a faster pace than we’ve ever seen before,” said Joseph. “The challenge is the educational institutions are still teaching the way they taught 20 years ago. Grads are walking into operations as instrumentation technologists and they are lost.”
Related: As more performance and control technologies are added to the processing plant, the measure of what is possible continues to grow
The mining industry is at a breakthrough moment in terms of predictive analytics technologies, said Dwyer, and with that will come changes. “I think there needs to be people in the industry who are able to marry the mechanical and computer analytics together,” she said.
On the other hand, added Dwyer, “sometimes it’s pretty hard to find a really good qualified mechanic for a mine to operate at maximum efficiency. I think if you can leverage the knowledge of a master mechanic and make it accessible to anyone, that will be a big win for the future.”
The future
Goldcorp has embarked on a growth and innovation journey, partnering with key technology and mining suppliers and service providers “to accelerate the pace of innovation and enable our mines to achieve our strategic objectives,” said Canepari.
In the not-too-distant future, however, he said he envisions hundreds of thousands of sensors at Goldcorp connected in real time through its systems, and a team of maintenance schedulers and reliability engineers enabled by the new technologies. “They will analyze all the data from a data science perspective as well as from a mechanical perspective. They will be looking for patterns, so we will be able to predict when an equipment is going to fail, not only from the equipment sensors but also all the other aspects, such as how the operator is driving it, the environment and integrated weather data,” said Canepari. “And how a machine is behaving in a particular mine and affected by all these factors benchmarking it against all our mines. Once you have all the holistic data with the computer power we have today, the sky’s the limit.”