It is widely recognized that operations and maintenance represent more than 70 per cent of life-cycle cost for equipment in the mining and processing industries. Maintenance is often performed on a schedule – annually, by distance or by hours – instead of being based on the actual state of health of the asset. Assets can receive more or less maintenance than needed, resulting in unnecessary costs and reduced reliability.
By combining engineering knowledge with machine learning, powerful hybrid models can be built that help companies understand the remaining useful life of physical assets and improve overall asset lifecycle management, even when the available data is imperfect.
Such a hybrid approach includes:
Engineering. Rather than relying solely on recognizing patterns from historical data, engineering analysis can provide key insights into an asset’s performance and deterioration. The engineering analyses used to extract information can include modelling and simulation, finite element analysis, durability analysis (physics of failure), noise and vibration analysis and reliability modelling.
Machine learning and data analytics. Measured historical data from the asset, combined with inspection/failure/repair information, is used to identify uncharacteristic asset behaviour and continuously improve and refine the asset deterioration model to predict asset health and performance.
Inferential sensing. Data that is easily obtainable using low-cost sensors can help to explain asset behaviour and to calculate the deterioration of critical components based on understanding physics of failure. The key is to identify and convert the “right” low-cost data into high-value actionable information. For example, the right low-cost data can be engine speed, oil temperature and other easily obtained vehicle bus measurements to calculate engine efficiency, which can be used to infer deteriorating condition over time.
Typically, predicting maintenance requirements for structural assets can be challenging, even though failure can lead to serious consequences because it often requires stoppages to conduct costly inspections. Lack of easy physical access can also make continuous monitoring difficult.
Related: Preparing for the coming demand for specialty minerals and metals for the battery revolution
This is where a “digital twin” of the asset can help. A recent initiative by Spectris Advance on inland waterways, which is highly applicable to the structural assets of the mining industry, such as tailings dams and water management facilities, demonstrated the effectiveness of using a digital twin in a hybrid approach. The asset degradation process of the waterway gates involved gaps being created between the gate and the wall, causing increased stresses that could finally collapse the gate.
Applying the hybrid approach, existing sensors (inferential sensing) on the gate were used to collect strain data. A representative finite element model of the gate was built (engineering) to understand strain distribution under different conditions. The model was validated against actual strain measurements and then run to generate new data and simulate different scenarios. This data was used to build the gate’s digital twin, correlating strain measurements with gap length considering associated uncertainty (e.g., temperature, water level) using machine learning. An accuracy of 96 per cent was achieved between model prediction and physical asset.
An initial deterioration model, built based on historical inspection and maintenance data showcasing average behaviour across multiple gates, is now continuously being updated as new strain data is collected from the field for each specific gate and its remaining useful life is calculated to enhance decision making (e.g., focus and allocation of maintenance budget, plus closure timing to minimize operational costs).
Aside from cost savings, one of the biggest benefits of this hybrid approach is that companies can still take advantage of all their available historical asset data to enhance asset management practices. Such an approach helps to use existing data first, even if not perfect, before considering investing more into data collection. It also minimizes the amount of data required for predictive analytics by applying engineering principles to create calculated metrics and increase the value of the existing data.
By using a hybrid approach that combines engineering with machine learning, miners can better predict performance, reduce failures, improve safety, optimize production and reduce operating costs for an average mine by as much as eight to 10 per cent without a huge investment in upfront data collection.
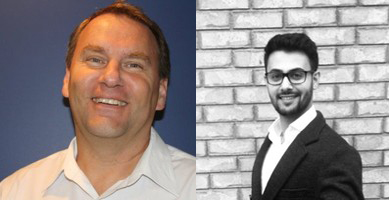
With contributions from Dr. Kevin Knill and Adi Dhora
Dr. Vasileios Geroulas, engineering solutions consultant, has expertise in reliability, vibration, fatigue and machine learning. Dr. Kevin Knill, director of solutions architecture, has expertise in developing analytical applications for heavy industries. Adi Dhora specializes in data-driven reliability methods for mining and metals operations. All are part of the Spectris Advance organization, a collection of Spectris companies delivering monitoring and analytics solutions for mining applications.