Courtesy of Guy Desharnais
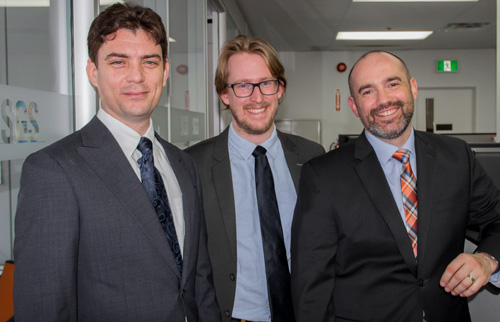
Left to right: Doug Hatfield, Jean-Phillippe Paiement and Guy Desharnais | Courtesy of Guy Desharnais
When the team at Quebec-based geological consulting firm SGS Geostat started brainstorming its entry for Integra Gold’s Gold Rush Challenge crowdfunding competition, the first idea was based on an existing concept: build a framework in which all available information would be integrated, interpolated and extrapolated. “We started off with a more conventional approach,” said Desharnais, technical manager of geological services in SGS’s minerals division. “We wanted to make sure we leveraged the data to the maximum, and populate a block model.” It was not until near the end of the process that the team came upon a more novel approach of machine learning.
On March 6 at the PDAC convention in Toronto, SGS Geostat won the $500,000 first prize at the competition intended to identify the best drilling targets on Integra’s Sigma-Lamaque property. By combining 3D geological interpretations with machine learning, SGS Geostat made a case for integrating artificial intelligence (AI) with more conventional exploration methodologies.
The team first used sophisticated geostatistical methods to push all the available data on the property into an expansive block model. Mineralization vectors were then combined to generate an overall prospectivity score between 0 and 100 for every block that covered the property to a depth of 2 kilometres. In parallel, the data was processed with an advanced machine learning algorithm to assist in identifying new targets and confirming the first set of scores. The prospectivity scoring system thus took into account both geological knowledge and machine learning. All of the geological data including the high-value targets were integrated into a virtual reality model using Oculus Rift technology for vetting and for the judges to examine.
Desharnais and SGS geologist Jean-Philippe Paiement originally came up with their own geological system, but they also understood the limitations of human reasoning. “Humans are biased by our very nature,” said Desharnais. “Jean-Philippe and I have a perceived understanding of what the controls are on ore gold mineralization, but we are biased based on our experience. So we decided to let the machine take a whack at this and try to do better than we had done.”
Desharnais started looking inside the SGS Canada network for people who would be inclined to have a look at the block model. He eventually contacted Doug Hatfield in Toronto. “Doug was already using quite sophisticated math concepts to do simulation work for geometallurgy, and I knew he was pushing the limits of the capabilities of math for other things,” said Desharnais. “He has a side interest in the technology.”
This was the first time SGS Canada applied machine learning to geoscience and mining. However, it could be part of its toolkit in the future. “Since winning the contest, we have had quite a bit of interest in applying our methodology to other properties,” said Desharnais.